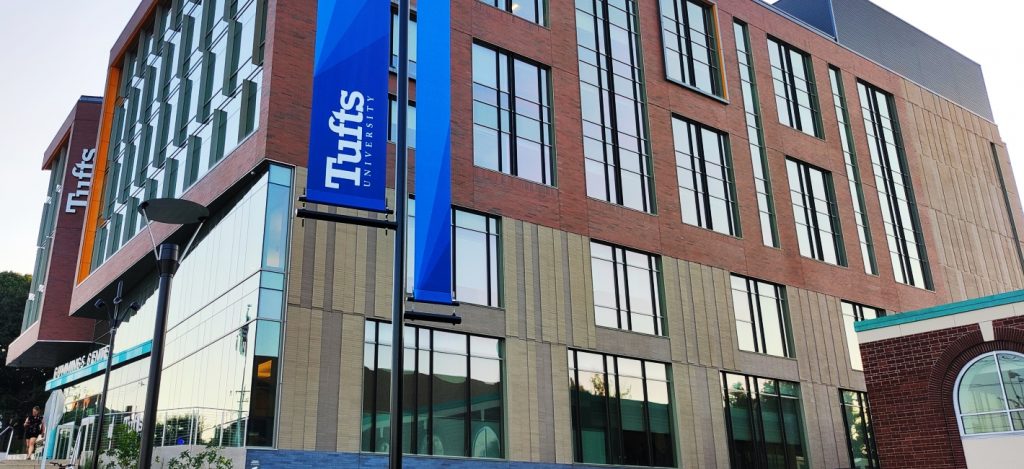
The beginning of the academic year in the United States coincided with a speech by Dr. Włodzimierz Lewoniewski at Tufts University. The colloquium discussed issues related to the use of artificial intelligence and large open data sets to automate the process of assessing the quality of Wikipedia articles and its information sources in various language versions.
The event took place on September 7, 2023 at the Joyce Cummings Center (JCC) during six-week visit of Dr. Włodzimierz Lewoniewski to the United States. This is the first colloquium (discussion seminar) at Tufts University in the 2023/2024 academic year. More information about seminar series with guest speakers discussing research challenges and recent advances in computer science can be found on the website of the Department of Computer Science at Tufts University.
Automatic assessment of Wikipedia quality
Wikipedia is one of the largest sources of information in the world with millions of articles in many languages. This encyclopedia offers free and open access to a huge amount of information on virtually any topic. Thanks to this, people all over the world can gain knowledge that they had no opportunity to learn before. Additionally, the content from this publicly available encyclopedia helps improve various websites (e.g. Google Search, ChatGPT, Facebook etc.).
Wikipedia is created by volunteers from all over the world, which makes it dynamic and constantly evolving. This collaboration model allows for quick updates and corrections of information. More than half a million new editions are made to this encyclopedia every day. Manually assessing all these changes in real time is a major challenge.
Wikipedia has certain standards for assessing the quality of content. However, the evaluation criteria may vary depending on the language version and may change over time. Moreover, assessing the quality of information is largely a subjective process, depending on the interpretation and experience of individual editors of this encyclopedia. Therefore, evaluating Wikipedia articles often requires dialogue and consensus among the community.
Automating the process of assessing the quality of Wikipedia’s information can significantly contribute to improving the quality of content, the efficiency of editors’ work and the credibility of the platform as a whole. Algorithms that are well designed have no emotion, bias or bias, which can help provide a more objective assessment of information quality. Additionally, automation allows for a uniform and consistent assessment of the quality of articles based on established criteria, which contributes to greater consistency in content assessment. Thanks to automation, large amounts of information quality data can also be collected and analyzed, which can provide valuable tips on areas requiring improvement and directions for further development of the platform. Additionally, automation can help relieve Wikipedia users from routine tasks, allowing them to focus on more complex aspects of editing and moderation.
Specially prepared tools can immediately identify potential problems, such as vandalism, inappropriate content or disinformation, which allows for faster response and improvement of content quality. These tools can provide editors with valuable real-time feedback, helping them create and edit articles according to Wikipedia’s guidelines. Additionally, automatic rating systems for Wikipedia articles and its information sources can be integrated with other tools and platforms, allowing for better use of technology to improve the quality of content. For example, WikiRank for quality assessment of Wikipedia articles, BestRef for evaluation of the information sources, and other publicly available web services.
It’s also important to remember that the Wikipedia community is made up of many volunteers who typically manually review and correct content. In the event of significant activity towards posting false information or mass vandalism, automatic tools can serve as the first line of defense, quickly identifying and reacting to unwanted changes.
A key aspect of content quality on Wikipedia is the principle of information verifiability. This means that every claim in the articles in this encyclopedia must be based on a reliable source of information. Automating the source evaluation process can help quickly identify sources that are potentially unreliable, outdated, or that do not meet academic standards, allowing editors to focus on verifying them or replacing them with more credible sources. Additionally, in times of increasing fake news, automatic source assessment can quickly detect and flag information based on questionable sources, preventing their spread. Additionally, new Wikipedia editors may not be sure which sources are the most reliable in a given field. Automatic source evaluation can provide them with guidance and recommendations, helping them select appropriate information source.
DBpedia and Wikidata
The presentation also presented some of the possibilities of open semantic knowledge bases that are closely related to Wikipedia – DBpedia and Wikidata. While DBpedia focuses on extracting data from Wikipedia into a more machine-friendly form, Wikidata serves as a central database supporting all Wikimedia projects in various languages. Together, these initiatives contribute to increasing access to knowledge in a more structured way. Improving quality on Wikipedia can contribute to improving these semantic knowledge bases.
Wikipedia, Wikidata and DBpedia are open resources that allow their content to be used for a variety of purposes. Better quality of these resources may contribute to the improvement of other services that use open data. Below is a list of examples of websites and applications that can use Wikipedia, DBpedia and Wikidata:
- Internet search engines: indexing and integrating content from these databases to improve search results.
- Semantic search engines: creating search engines that understand the context of a query using structured data from DBpedia or Wikidata.
- Natural language processing (NLP): using content to train language models or for syntactic analysis.
- Educational applications: using content to create teaching materials. For example, the application uses Wikipedia articles to present the user with an interactive timeline of important historical events, while enabling deeper knowledge through links to full entries.
- Recommendation systems: can use data from these sources to recommend articles or related topics. For example, by analyzing user preferences, the system suggests movies (or games, books, etc.) based on actors, directors or genres, using information from DBpedia or Wikidata, and then offers links to related entries on Wikipedia for a deeper understanding of the context. li>
- Creating educational games: using data to create quizzes, board games or computer games with questions based on content from these databases.
- Developing thematic stories: for example, educational paths or tourist trips based on content from Wikipedia.
- Knowledge clouds and ontologies: for creating semantic knowledge bases. For example, corporations can use data from DBpedia and Wikidata to create personalized knowledge clouds that integrate industry information with general knowledge, enabling employees to quickly access consistent and up-to-date data.
- Virtual assistants and chatbots: can use these sources to provide answers to users’ questions. For example, a virtual assistant uses ontologies from DBpedia to understand the semantic connections between different topics, which allows for more fluid and contextually rich interaction with the user.
- Data analysis services: analyze and visualize data from these sources. For example, such websites can use Wikipedia’s editing history to monitor and analyze the most frequently updated topics, which may indicate growing interest in a given event or topic in the world.
- Network analysis: Using DBpedia and Wikidata, websites can create networks of connections between different entities (e.g. people, places, events), which allows for a deeper understanding of relationships and patterns occurring in complex sets data.
- Language learning apps: using content to create learning materials for different languages. For example, users can be presented with Wikipedia articles in two languages simultaneously, enabling comparison of linguistic structures and better understanding of the translation context.
- Research: Researchers can use this data to analyze, investigate and create new knowledge. For example, using DBpedia and Wikidata to create specialized semantic databases that help in the analysis and interpretation of complex sets of information, for example in molecular biology or social sciences.
- Creating maps and geolocation applications: using geographic and historical data to create interactive maps.
- Cultural and tourism applications: may present information about places, people or historical events.
- Integration with AR/VR applications: use of data for virtual or augmented reality applications that can provide information about the user’s surroundings.
- Sentiment analysis: Using article edit history to analyze sentiment in discussions on various topics. For example, you can track changing opinions about controversial topics or figures by seeing how the wording and tone of articles evolve in response to current events.
- Data linking: combining data from these databases with other open sources to create richer sets of information.
- Content personalization: applications or websites can adapt content based on Wikipedia to the individual needs and interests of users.